1. Kafka Connector
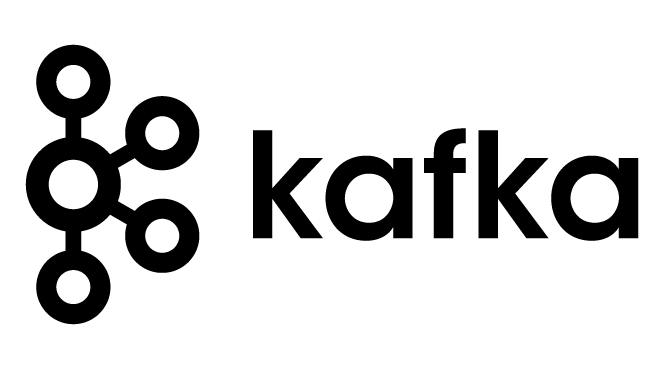
1.1. Concepts
Kafka is a messaging system based on the producer-consumer pattern that uses internal data structures, called topics, which temporarily store received data until someone subscribes (i.e., connects) to consume the stored data. Kafka is considered a persistent, scalable, replicated, and fault-tolerant system. In addition, it offers good read and write speeds, making it an excellent tool for streaming communications.
1.1.1. Key Concepts
Kafka Server
As a server that manages topics, Kafka listens via an IP and port. Therefore, we must boot it into the operating system with a process that corresponds to the server, such as Tomcat or JBoss. When we connect to the server to place records into a topic or consume topic records, we simply specify the name of the topic along with the IP and port on which Kafka’s server listens.
Topics, producers and consumers
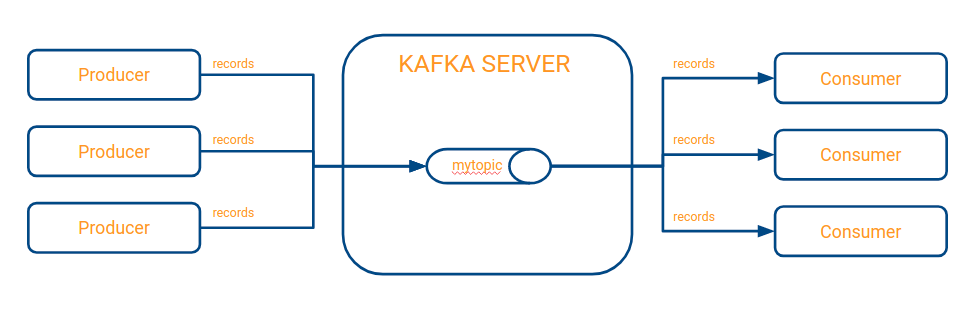
This picture illustrates the main idea of the Kafka server. A topic within Kafka acts as a FIFO tail for which one or more producers can send records (e.g., messages) to a topic, and one or more consumers can read from a topic. Consumers always read the records in the order in which they were inserted, and records remain available to all consumers, even if one has consumed it previously. This feature is achieved by keeping track of the offset per consumer, which is a sequential number that locates the last record read from a topic and is unique per consumer. With this approach, a record is ensured that if one consumer has already read it, then it remains available to all other consumers who need it because they will have a different offset from the one that previously consumed it.
If we send a record into Kafka as a producer, then libraries exist for nearly all programming languages. In Java, the following is a basic, partial example to illustrate the idea:
import org.apache.avro.generic.GenericRecord;
import org.apache.kafka.clients.producer.KafkaProducer;
import org.apache.kafka.clients.producer.ProducerConfig;
import org.apache.kafka.clients.producer.ProducerRecord;
import org.apache.kafka.clients.producer.RecordMetadata;
// Create properties configuration file
Properties props = new Properties();
// Add Kafka server ip and port to configuration
props.put(ProducerConfig.BOOTSTRAP_SERVERS_CONFIG,"localhost:9092");
// Create producer
KafkaProducer producer = new KafkaProducer(props);
// Create record
// Create record key
GenericRecord recordKey = new GenericRecord();
// Here would be the rest of statements to fill in the record key
// Create record value
GenericRecord recordvalue = new GenericRecord();
// Here would be the rest of statements to fill in the record value
// Create record producer for topic
ProducerRecord<Object, Object> record = new ProducerRecord<>("mytopic", recordKey, recordValue);
// Send record
RecordMetadata res = producer.send(record);
On the other hand, if we want to read from Kafka as a consumer, then a basic example is:
import org.apache.kafka.clients.consumer.KafkaConsumer;
import org.apache.kafka.clients.consumer.ConsumerConfig;
import org.apache.kafka.clients.consumer.ConsumerRecords;
// Create properties configuration file
Properties props = new Properties();
// Add Kafka server ip and port to configuration
props.put(ConsumerConfig.BOOTSTRAP_SERVERS_CONFIG, "localhost:9092");
// Create consumer
KafkaConsumer consumer = new KafkaConsumer(props);
//Subscribe consumer
consumer.subscribe(Collections.singletonList(“mytopic”));
// Get 1000 records from the topic
ConsumerRecords<Long, String> consumerRecords = consumer.poll(1000);
Connectors
The above example demonstrates how to connect to the Kafka server to produce and consume records. However, these approaches can only be accomplished if we have control over the code of the applications inserting or reading records from Kafka’s topics. If the source of our data is a database, then we will not have control over the code of that database because it is a proprietary product with a proprietary life cycle. For this scenario, connectors are available. A Kafka connector is a separate process from the Kafka server that acts as a proxy between the data sources and the Kafka server.
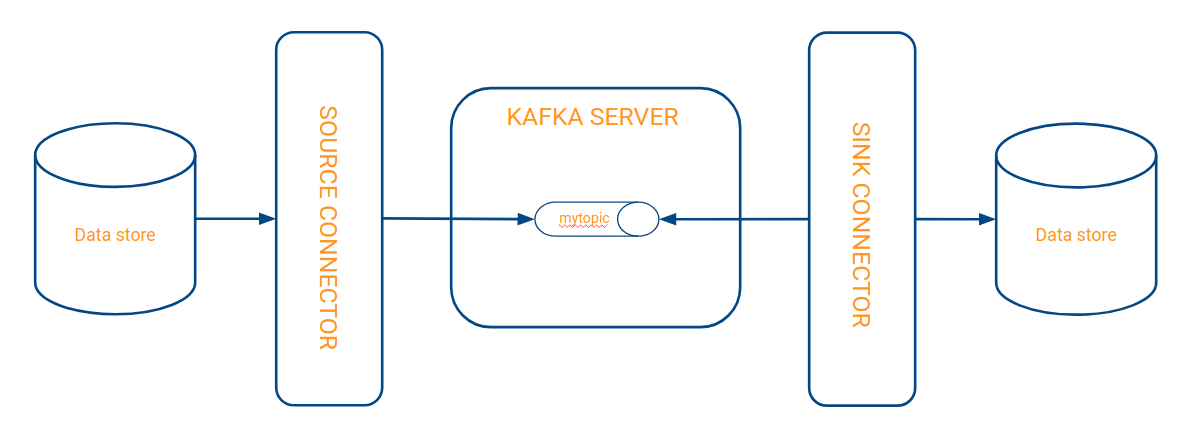
As seen in the diagram, the connectors are intermediaries between the data sources, such as a database, and the Kafka server. In this example, we have a source database from which a raised connector reads as well as inserts topics into Kafka’s server. A second raised connector reads from Kafka’s topics and inserts these into another destination database. Predefined, open source connectors are already available to anyone to access, such as the generic JDBC connector, file connectors, Amazon S3 loop connectors, and many more for NoSQL databases, like MongoDB. LeanXcale also features a Kafka sink and source connectors.
The Kafka connector for LeanXcale uses the direct NoSQL API to insert data. The direct API, as discussed in previous posts, is a MongoDB-style NoSQL interface for inserts, queries, and all operations executable by JDBC. This approach is much faster than using SQL and is tremendously powerful for inserting large amounts of data into LeanXcale.
1.1.2. Additional Concepts
A few additional concepts must be considered to understand the overall functioning of a Kafka architecture.
Data serialization
The data contained in Kafka’s topics are neither text nor readable objects or entities. Instead, they are key-value structures with byte string values. Serializers are then needed to provide the data meaning. A data serializer is an entity that collects byte strings and transforms them into a readable format for a system to use, such as a JSON string or Java object. Several types of serializers are available, for example:
-
StringSerializer: transforms data bytes into simple strings, or strings into data bytes.
-
IntegerSerializer: transforms data bytes into whole numbers or whole numbers into data bytes. In the same way, the types Long, Integer, and Double each have serializers.
-
JSONSerializer: transforms data bytes into JSON strings or JSON strings into data bytes. JSON is a framework for serializing logical objects into strings with a predefined format.
-
AvroSerializer: transforms data bytes into logical objects with the special feature that it can determine the format of the data that it serializes and deserializes because of how it works with the data schemas it serializes. Avro is the most used serializer, so a more detailed explanation is provided below.
The implementation of the serializers is automatic from the perspective of the programmer, who only needs to leverage specially designed libraries. The Kafka connector for LeanXcale is also automatic, and the serializer is configured within a properties file. The inclusion of the serializers into an architecture diagram with a producer and a sink connector is illustrated as follows:
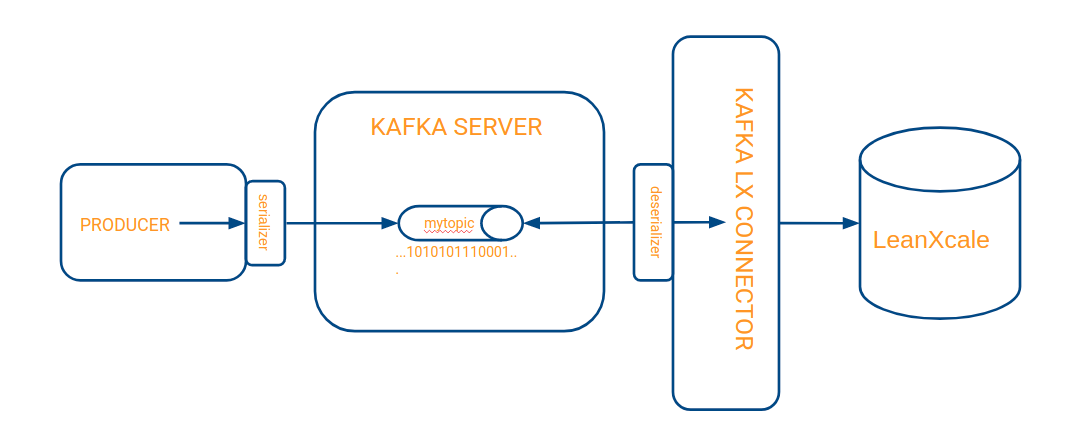
The serializer code is embedded in the producer and is executed just before sending data to the topic so that it receives the data already serialized. Similarly, the deserializer is embedded in the connector and runs just before passing the data to the connector code, so that it reads the bytes of the topic and transforms them into something understandable by the connector code.
Schemas
Before converted into a string of bytes, a record can obey a specified schema. The Kafka LeanXcale connector requires that the registers it receives follow a scheme predefined by the programmer. For example, to send records with three fields of the ID, first name, and last name of a person, an example of the defined schema is:
{
"type":"struct",
"fields":[
{
"type":"int64",
"optional":false,
"field":"id"
},
{
"type":"string",
"optional":false,
"field":"name"
},
{
"type":"string",
"optional":false,
"field":"surname"
}
],
"optional":false,
"name":"record1"
}
This schema defines the format of the records, which is represented in JSON format and contains fields for the ID, which is a long data type, the name as a string, and a surname as another string. The Kafka connector for LeanXcale requires records to obey a schema through the use of its DDLs execution capabilities to control the structure of the target tables. In other words, if a record is received that obeys the previous schema and the automatic creation option is enabled, then the connector creates the target table with the columns corresponding to the fields specified in the schema.
Avro and schemas
As introduced above, the specific serializer and deserializer most commonly used is Avro because it always works with the schemas of the data it serializes. Because Kafka’s connector for LeanXcale requires a schema, this type of serialization is a good option for this scenario because it ensures that schemas exist and are configured as expected. When Avro reads or writes a byte string, the schema applied to serialize the data is always present. In this case, when the Avro serializer converts the record created by the Java producer into a byte string, it automatically registers the provided schema, so that it is retrieved when the record reaches the connector. This feature allows to offload the Kafka topic of redundant information. Otherwise, if we did not follow this procedure with Avro, then sending the schema in JSON format would be required for each record sent to the topic. Instead, the schema is registered one time, and only the specified field values are sent to the topics. The following is an example of a complete record:
{
"schema":{
"type":"struct",
"fields":[
{
"type":"int64",
"optional":false,
"field":"id"
},
{
"type":"string",
"optional":false,
"field":"name"
},
{
"type":"string",
"optional":false,
"field":"surname"
}
],
"optional":false,
"name":"record1"
},
"payload":{
"id":1,
"name":"Renata",
"surname":"Mondongo"
}
}
This record consists of the primary fields "schema" and "payload." The schema field is of the type struct (i.e., an object) and contains the desired fields. The payload field contains the values of the defined fields from the schema. Sending another record without Avro as the serializer requires sending all the content again, as in:
{
"schema":{
"type":"struct",
"fields":[
{
"type":"int64",
"optional":false,
"field":"id"
},
{
"type":"string",
"optional":false,
"field":"name"
},
{
"type":"string",
"optional":false,
"field":"surname"
}
],
"optional":false,
"name":"record1"
},
"payload":{
"id":2,
"name":"Renata",
"surname":"Porrongo"
}
}
However, with Avro, the schema is sent only once, and all subsequent records are also sent:
{ "id": 2,"name": "Renata","surname":"Porrongo"}
Schema registry
For the Avro serializer to register and retrieve the schema as described above, a new component must be introduced, called the schema registry, which is another process distinct from the Kafka server and LeanXcale connector. The final architecture for this scenario becomes:
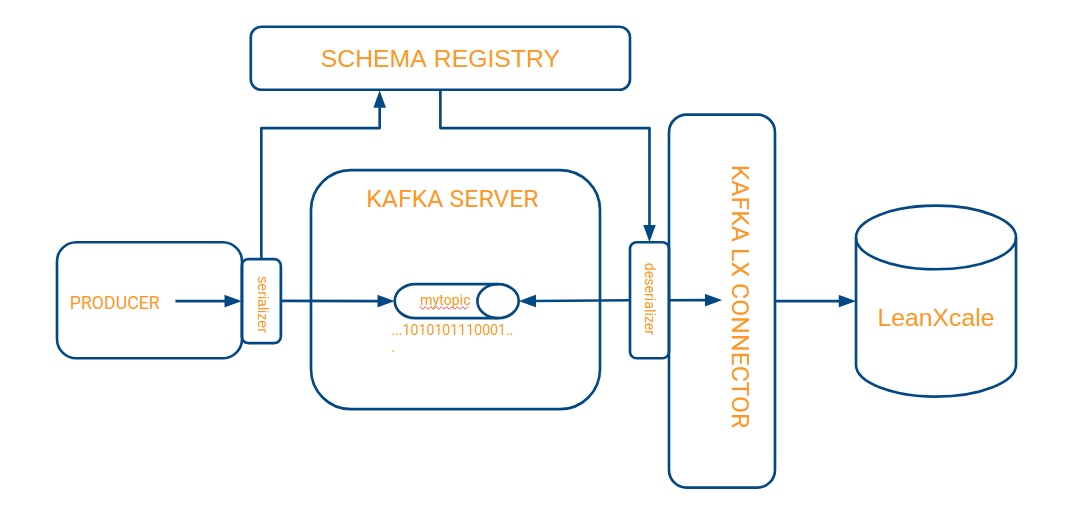
The complete flow of information is processed as the following:
-
The Java producer creates a record for Kafka and transmits that the selected serializer is Avro.
-
The producer executes a send of the record to Kafka.
-
Before records arrive at Kafka, the Avro serializer stores the specified schema in the registry schema and converts it into a byte sequence.
-
This byte sequence is sent to Kafka’s topic, called "mytopic."
-
Kafka’s connector queries Kafka to retrieve the stored records.
-
Before the connector receives the records, the Avro deserializer connects to the registry schema and validates the records collected from Kafka against the schema, and converts the byte string representing the record into a Java object.
-
The connector determines if there a table already exists in the target LeanXcale that complies with the registry schema. When one is not found, it creates the table automatically and includes the fields defined in the schema registry as columns.
-
The connector stores the records collected from Kafka in the new LeanXcale table.
Zookeeper
Appreciating that the execution of even this simple example, three distinct processes are required, which can also execute in parallel. So, the Kafka suite relies on a centralized process manager, such as Zookeeper, to maintain fast and efficient access to each of these processes. Therefore, to run the Kafka server architecture, registry schema, and LeanXcale connector, a Zookeeper application must be previously initiated that is responsible for maintaining and managing the communication between the three processes involved in the distribution of records. In practice, Zookeeper is responsible for much more. The Kafka suite also allows for defining a clusterization of its components, so, in this case, Zookeeper further manages the processes that comprise the cluster.
1.2. Installation
To implement the general architecture, the Kafka suite must first be downloaded. Confluent is an open source distribution by Kafka, founded by the original creators of Kafka, and offers a set of tools and utilities related to the complete management of the server. Specifically, this package includes Zookeeper, Kafka Server, Schema Registry, and Kafka Connect, which covers all the components needed to execute.
The Kafka suite can be downloaded from here.
If we untar the file, the following directory structure should remain:
Folder |
Description |
/bin/ |
Driver scripts for starting and stopping services |
/etc/ |
Configuration files |
/lib/ |
System services |
/logs/ |
Log files |
/share/ |
JARS and licenses |
/src/ |
Source files that require a platform-dependent build |
1.2.1. Configuration
Please note the Kafka configuration described in this documentation is the default and simplest one, with just one broker.
Zookeeper
Navigate to the confluent-5.4.1/etc/kafka
directory and open the zookeeper.properties
file. Leave the default configuration
in place, and make sure the file contains:
clientPort=2181
This is the port to listen for client connections.
Kafka server
Navigate to the confluent-5.4.1/etc/kafka
directory and open the server.properties
file. Leave the default configuration,
and make sure the file contains:
broker.id=0
listeners=PLAINTEXT://:9092
With these lines, the broker’s identifier is defined (i.e., the server, where this example includes only one, but more servers could act in a cluster) as well as the port where Kafka listens (i.e., 9092).
To run the client from a different machine from the kafka server, we must set up a reachable server ip (localhost/0.0.0.0 not valid for remote connections)
listeners=PLAINTEXT://ip_kafka_server:9092
Schema registry
Navigate to the confluent-5.4.1/etc/schema-registry
directory and open the schema-registry.properties
file. Leave the
default configuration, and make sure the file contains:
listeners=http://0.0.0.0:8081
This line defines the IP address and port where the schema registry listens, and the default value is 8081.
Kafka connect
Navigate to the confluent-5.4.1/etc/kafka
directory and open the connect-standalone.properties
file. The following lines
must exist:
bootstrap.servers=0.0.0.0:9092 // Kafka server ip and port
group.id=mytopic // Consumer group id
max.poll.interval.ms=300000 // The maximum delay between invocations of poll()
consumer.max.poll.records=10000 // The maximum number of records returned in a single call to poll().
offset.flush.interval.ms=50000 // Maximum number of milliseconds to wait for records to flush and partition offset data
rest.port=8083 // Port for the connect REST API to listen on.
plugin.path=/usr/local/share/kafka/plugins // Kafka Connect plugin directory
Kafka connector for LeanXcale
Download the Kafka connector for LeanXcale from the Drivers page.
The connector is contained in a tar.gz, so it must be unpacked into the directory indicated as plugin.path in the Kafka connect configuration.
Once extracted, the connector has to be configured. In case you are running a sink connector, navigate to the directory confluent-5.4.1/etc/kafka
and create a new file
named "connect-lx-sink.properties" with the following example configuration added to the file:
name=local-lx-sink // Name of the connector
connector.class=com.leanxcale.connector.kafka.LXSinkConnector // Java class executed when the connector is lifted
tasks.max=1 // Number of threads that read from the topic
topics=mytopic // Name of the topic to read from
connection.url=lx://ip_bd_leanxcale:9876 // LeanXcale URL
connection.user=APP // LeanXcale user
connection.password=APP // LeanXcale password
connection.database=mytestdatabase // LeanXcale database
auto.create=true // If true, the connector should create the target table if not found
delete.enabled=true // If deletion is enabled.
insert.mode=insert // Insertion mode. May be insert, upsert or update.
batch.size=500 // Maximum number of records to be sent in a single commit to LeanXcale
connection.check.timeout=20 // Active connection checkout time
// Key and value converters according to the architecture and with the schema registry url
key.converter=io.confluent.connect.avro.AvroConverter
key.converter.schema.registry.url=http://localhost:8081
value.converter=io.confluent.connect.avro.AvroConverter
value.converter.schema.registry.url=http://localhost:8081
sink.connection.mode=kivi // LeanXcale connection mode value that defines the connection through the NoSQL interface
sink.transactional=false // Whether the load on LeanXcale is going to be executed with ACID transactions or not.
// This scenario potentially increases the insertion performance, and is indicated in case of big initial loads without
consistency requirements over operational environments.
sink.defer.timeout=10000 // Number of milliseconds to wait before closing the physical connection to LeanXcale
table.name.format=${topic} // Name of the destination table where ${topic} represents what it will be called as the topic.
pk.mode=record_key // Indicates the primary key mode of the target table where
// record_key means that it will use the key field of the record stored
// in Kafka as the primary key in the target table.
pk.fields=id // Fields of the pk among what arrives in the key field. In our
// case, only one exists in the "id" field.
fields.whitelist=field1,field2,field3 // List of the fields in the record to be
// columns in the target table. If all fields are included, then all will
// become columns. However, those that should not be created in the table can
// be removed.
In case you are running a source connector, navigate to the directory confluent-5.4.1/etc/kafka
and create a new file
named "connect-lx-source.properties" with the following example configuration added to the file:
#Source connector name
name=local-lx-source
#Java class executed when the connector is lifted
connector.class=com.leanxcale.connector.kafka.LXSourceConnector
#Number of threads started by kafka
tasks.max=1
#Key and value converters according to the architecture and with the schema registry url
key.converter=io.confluent.connect.avro.AvroConverter
key.converter.schema.registry.url=http://localhost:8081
value.converter=io.confluent.connect.avro.AvroConverter
value.converter.schema.registry.url=http://localhost:8081
#leanxcale connection URL
connection.properties=lx://localhost:9876/test@APP
#Source table to read data from and sent it to kafka
source.table=LX_SOURCE_TABLE
#Max size of the intermediate queue where read tuples are temporary stored
source.queue.size=10000
#Sets where the tuples will be read from. Available modes are: offset(tuples from last run), now(only new tuples), begin(all tuples)
source.from.mode=offset
#"Name of the topic where send the tuples. The pattern ${table} will be replaced by the name of the source table
source.topic.name=${table}
#If not empty, just fields from this list will be sent to kafka
source.fields.whitelist=
Security configuration
REVISAR SEGURIDAD TLS
If your LeanXcale instance is configuring with security, you will have to generate an application certificate in order to allow your Kafka connector to connect to LeanXcale. Learn how to do this
Once this is done, configure the connector to enable security and provide the path to the certificate file using this properties in the configuration file:
// Security properties
connection.security.enable=true
connection.security.ceth.path=/path/to/file.kcf
You can find more examples in subsequent provisions of this documentation, and also check Kafka official documentation in https://docs.confluent.io/current/
1.3. Execution
After everything above is installed and configured, the processes have to be started.
1.3.1. Zookeeper
The first step is to start the process manager, Zookeeper. Without this tool, no other component will start because they
rely on this to find one another. Navigate to the confluent-5.4.1/bin
directory and run:
nohup ./zookeeper-server-start ../etc/kafka/zookeeper.properties > nohup_zk.out &
Previewing the newly generated log with the command tail, the last few lines will including something like:
[2020-03-31 09:07:55,343] INFO Using org.apache.zookeeper.server.NIOServerCnxnFactory as server connection factory (org.apache.zookeeper.server.ServerCnxnFactory)
[2020-03-31 09:07:55,345] INFO Configuring NIO connection handler with 10s sessionless connection timeout, 2 selector thread(s), 24 worker threads, and 64 kB direct buffers. (org.apache.zookeeper.server.NIOServerCnxnFactory)
[2020-03-31 09:07:55,347] INFO binding to port 0.0.0.0/0.0.0.0:2181 (org.apache.zookeeper.server.NIOServerCnxnFactory)
[2020-03-31 09:07:55,357] INFO zookeeper.snapshotSizeFactor = 0.33 (org.apache.zookeeper.server.ZKDatabase)
[2020-03-31 09:07:55,359] INFO Snapshotting: 0x0 to /tmp/zookeeper/version-2/snapshot.0 (org.apache.zookeeper.server.persistence.FileTxnSnapLog)
[2020-03-31 09:07:55,361] INFO Snapshotting: 0x0 to /tmp/zookeeper/version-2/snapshot.0 (org.apache.zookeeper.server.persistence.FileTxnSnapLog)
[2020-03-31 09:07:55,372] INFO Using checkIntervalMs=60000 maxPerMinute=10000 (org.apache.zookeeper.server.ContainerManager)
1.3.2. Kafka server
After Zookeeper is started, the Kafka server is initiated from the confluent-5.4.1/bin
directory with the command:
nohup ./kafka-server-start ../etc/kafka/server.properties > nohup_kafka_server.out &
By tailing the log file with tail, something like the following will be displayed:
[2020-03-31 09:11:44,434] INFO Kafka version: 5.4.1-ccs (org.apache.kafka.common.utils.AppInfoParser)
[2020-03-31 09:11:44,435] INFO Kafka commitId: 1c8f62230319e789 (org.apache.kafka.common.utils.AppInfoParser)
[2020-03-31 09:11:44,435] INFO Kafka startTimeMs: 1585638704434 (org.apache.kafka.common.utils.AppInfoParser)
[2020-03-31 09:11:44,455] INFO [Producer clientId=producer-1] Cluster ID: _KCRiquMRVm85wsVsE3ATQ (org.apache.kafka.clients.Metadata)
[2020-03-31 09:11:44,497] INFO [Producer clientId=producer-1] Closing the Kafka producer with timeoutMillis = 9223372036854775807 ms. (org.apache.kafka.clients.producer.KafkaProducer)
[2020-03-31 09:11:44,499] INFO Successfully submitted metrics to Kafka topic __confluent.support.metrics (io.confluent.support.metrics.submitters.KafkaSubmitter)
[2020-03-31 09:11:45,815] INFO Successfully submitted metrics to Confluent via secure endpoint (io.confluent.support.metrics.submitters.ConfluentSubmitter)
1.3.3. Schema registry
After the Kafka server starts, the registry schema is initiated so that Avro registers the schema of the records it sends.
From confluent-5.4.1/bin
, launch the command:
nohup ./schema-registry-start ../etc/schema-registry/schema-registry.properties > nohup_schema_registry_server.out &
Check the status with tail:
[2020-03-31 09:14:39,120] INFO HV000001: Hibernate Validator 6.0.11.Final (org.hibernate.validator.internal.util.Version:21)
[2020-03-31 09:14:39,283] INFO JVM Runtime does not support Modules (org.eclipse.jetty.util.TypeUtil:201)
[2020-03-31 09:14:39,283] INFO Started o.e.j.s.ServletContextHandler@66b7550d{/,null,AVAILABLE} (org.eclipse.jetty.server.handler.ContextHandler:824)
[2020-03-31 09:14:39,294] INFO Started o.e.j.s.ServletContextHandler@2ccca26f{/ws,null,AVAILABLE} (org.eclipse.jetty.server.handler.ContextHandler:824)
[2020-03-31 09:14:39,305] INFO Started NetworkTrafficServerConnector@3e57cd70{HTTP/1.1,[http/1.1]}{0.0.0.0:8081} (org.eclipse.jetty.server.AbstractConnector:293)
[2020-03-31 09:14:39,305] INFO Started @2420ms (org.eclipse.jetty.server.Server:399)
[2020-03-31 09:14:39,305] INFO Server started, listening for requests... (io.confluent.kafka.schemaregistry.rest.SchemaRegistryMain:44)
1.3.4. Kafka Sink Connector for LeanXcale
Finally, the Kafka Sink connector for LeanXcale is initiated. Execute the following command:
nohup ./connect-standalone ../etc/kafka/connect-standalone.properties ../etc/kafka/connect-lx-sink.properties > nohup_connect_lx.out &
Looking at the log:
[2020-03-31 09:19:49,235] INFO [Consumer clientId=connector-consumer-local-jdbc-sink-0, groupId=connect-local-jdbc-sink] (Re-)joining group (org.apache.kafka.clients.consumer.internals.AbstractCoordinator:533)
[2020-03-31 09:19:49,285] INFO [Consumer clientId=connector-consumer-local-jdbc-sink-0, groupId=connect-local-jdbc-sink] (Re-)joining group (org.apache.kafka.clients.consumer.internals.AbstractCoordinator:533)
[2020-03-31 09:19:49,305] INFO [Consumer clientId=connector-consumer-local-jdbc-sink-0, groupId=connect-local-jdbc-sink] Finished assignment for group at generation 1: {connector-consumer-local-jdbc-sink-0-ae0d5fda-a1f2-4a10-95ca-23bd3246481c=Assignment(partitions=[mytopic-0])} (org.apache.kafka.clients.consumer.internals.ConsumerCoordinator:585)
[2020-03-31 09:19:49,321] INFO [Consumer clientId=connector-consumer-local-jdbc-sink-0, groupId=connect-local-jdbc-sink] Successfully joined group with generation 1 (org.apache.kafka.clients.consumer.internals.AbstractCoordinator:484)
[2020-03-31 09:19:49,324] INFO [Consumer clientId=connector-consumer-local-jdbc-sink-0, groupId=connect-local-jdbc-sink] Adding newly assigned partitions: mytopic-0 (org.apache.kafka.clients.consumer.internals.ConsumerCoordinator:267)
[2020-03-31 09:19:49,337] INFO [Consumer clientId=connector-consumer-local-jdbc-sink-0, groupId=connect-local-jdbc-sink] Found no committed offset for partition mytopic-0 (org.apache.kafka.clients.consumer.internals.ConsumerCoordinator:1241)
[2020-03-31 09:19:49,343] INFO [Consumer clientId=connector-consumer-local-jdbc-sink-0, groupId=connect-local-jdbc-sink] Resetting offset for partition mytopic-0 to offset 0. (org.apache.kafka.clients.consumer.internals.SubscriptionState:381)
If there are data on the topic, the connector will immediately start to consume the records and store them on LeanXcale according to the supplied configuration. We will find in the log file lines like these:
[2020-03-31 09:33:43,342] INFO Flushing records in writer for table com.leanxcale.connector.kafka.utils.metadata.TableId@a25226fa with name mytopic (com.leanxcale.connector.kafka.sink.impl.LXWriterImpl:54)
[2020-03-31 09:33:43,342] INFO Connecting to database for the first time... (com.leanxcale.connector.kafka.connection.impl.AbstractLXConnectionProvider:33)
[2020-03-31 09:33:43,342] INFO Attempting to open connection (com.leanxcale.connector.kafka.connection.impl.AbstractLXConnectionProvider:60)
Conn: loading libkv from libkv.so
Java HotSpot(TM) 64-Bit Server VM warning: You have loaded library /tmp/libkv41983156673418059.so which might have disabled stack guard. The VM will try to fix the stack guard now.
It's highly recommended that you fix the library with 'execstack -c <libfile>', or link it with '-z noexecstack'.
[2020-03-31 09:33:43,408] INFO Registering builder com.leanxcale.kivi.database.constraint.GeohashConstraintBuilder@355d0da4 for prefix GH (com.leanxcale.kivi.database.impl.TableMetadataSerializer:34)
[2020-03-31 09:33:43,409] INFO Registering builder com.leanxcale.kivi.database.constraint.DeltaConstraintBuilder@4d19b0e7 for prefix DEL (com.leanxcale.kivi.database.impl.TableMetadataSerializer:34)
[2020-03-31 09:33:43,410] INFO Registering builder com.leanxcale.kivi.database.constraint.UUIDConstraintBuilder@6033bc5c for prefix UUID (com.leanxcale.kivi.database.impl.TableMetadataSerializer:34)
[2020-03-31 09:33:43,411] INFO Registering builder com.leanxcale.kivi.database.constraint.ReflectStorableConstraint@3ff680bd for prefix ABS (com.leanxcale.kivi.database.impl.TableMetadataSerializer:34)
09:33:43 1585640023972632000 kv.Conn[26954]: *** no.auth = 1
09:33:43 1585640023972664000 kv.Conn[26954]: *** no.crypt = 2
09:33:43 1585640023972669000 kv.Conn[26954]: *** no.chkfull = 1
09:33:43 1585640023972673000 kv.Conn[26954]: *** no.flushctlout = 1
09:33:43 1585640023972677000 kv.Conn[26954]: *** no.npjit = 1
09:33:43 1585640023972681000 kv.Conn[26954]: *** no.tplfrag = 1
09:33:43 1585640023972684000 kv.Conn[26954]: *** no.dictsort = 1
[2020-03-31 09:33:44,346] INFO New session created: 1 (com.leanxcale.connector.kafka.connection.impl.LXKiviConnectionProvider:46)
[2020-03-31 09:33:44,346] INFO Table com.leanxcale.connector.kafka.utils.metadata.TableId@a25226fa is not registered for the connector. Checking db... (com.leanxcale.connector.kafka.sink.impl.LXWriterImpl:60)
[2020-03-31 09:33:44,347] INFO Table mytopic not found. Creating it (com.leanxcale.connector.kafka.sink.impl.LXWriterImpl:62)
[2020-03-31 09:33:44,453] INFO Commited session: 1 (com.leanxcale.connector.kafka.connection.impl.LXKiviConnectionProvider:78)
[2020-03-31 09:33:44,453] INFO Registering table mytopic in connector (com.leanxcale.connector.kafka.sink.impl.LXWriterImpl:71)
[2020-03-31 09:33:44,453] INFO Writing 1 records (com.leanxcale.connector.kafka.sink.impl.LXWriterImpl:84)
[2020-03-31 09:33:44,817] INFO Commited session: 1 (com.leanxcale.connector.kafka.connection.impl.LXKiviConnectionProvider:78)
[2020-03-31 09:33:44,821] INFO Flushing records in writer for table com.leanxcale.connector.kafka.utils.metadata.TableId@a25226fa with name mytopic (com.leanxcale.connector.kafka.sink.impl.LXWriterImpl:54)
[2020-03-31 09:33:44,821] INFO Connecting to database... (com.leanxcale.connector.kafka.connection.impl.AbstractLXConnectionProvider:36)
[2020-03-31 09:33:44,821] INFO Attempting to open connection (com.leanxcale.connector.kafka.connection.impl.AbstractLXConnectionProvider:60)
[2020-03-31 09:33:44,821] INFO New session created: 2 (com.leanxcale.connector.kafka.connection.impl.LXKiviConnectionProvider:46)
[2020-03-31 09:33:44,821] INFO Table mytopic is already registered for the connector (com.leanxcale.connector.kafka.sink.impl.LXWriterImpl:75)
[2020-03-31 09:33:44,821] INFO Writing 4 records (com.leanxcale.connector.kafka.sink.impl.LXWriterImpl:84)
[2020-03-31 09:33:45,004] INFO Commited session: 2 (com.leanxcale.connector.kafka.connection.impl.LXKiviConnectionProvider:78)
[2020-03-31 09:33:45,080] INFO Flushing records in writer for table com.leanxcale.connector.kafka.utils.metadata.TableId@a25226fa with name mytopic (com.leanxcale.connector.kafka.sink.impl.LXWriterImpl:54)
[2020-03-31 09:33:45,080] INFO Connecting to database... (com.leanxcale.connector.kafka.connection.impl.AbstractLXConnectionProvider:36)
[2020-03-31 09:33:45,080] INFO Attempting to open connection (com.leanxcale.connector.kafka.connection.impl.AbstractLXConnectionProvider:60)
[2020-03-31 09:33:45,080] INFO New session created: 3 (com.leanxcale.connector.kafka.connection.impl.LXKiviConnectionProvider:46)
[2020-03-31 09:33:45,080] INFO Table mytopic is already registered for the connector (com.leanxcale.connector.kafka.sink.impl.LXWriterImpl:75)
[2020-03-31 09:33:45,080] INFO Writing 500 records (com.leanxcale.connector.kafka.sink.impl.LXWriterImpl:84)
[2020-03-31 09:33:45,563] INFO Commited session: 3 (com.leanxcale.connector.kafka.connection.impl.LXKiviConnectionProvider:78)
[2020-03-31 09:33:45,603] INFO Flushing records in writer for table com.leanxcale.connector.kafka.utils.metadata.TableId@a25226fa with name mytopic (com.leanxcale.connector.kafka.sink.impl.LXWriterImpl:54)
[2020-03-31 09:33:45,604] INFO Connecting to database... (com.leanxcale.connector.kafka.connection.impl.AbstractLXConnectionProvider:36)
[2020-03-31 09:33:45,604] INFO Attempting to open connection (com.leanxcale.connector.kafka.connection.impl.AbstractLXConnectionProvider:60)
[2020-03-31 09:33:45,604] INFO New session created: 4 (com.leanxcale.connector.kafka.connection.impl.LXKiviConnectionProvider:46)
[2020-03-31 09:33:45,604] INFO Table mytopic is already registered for the connector (com.leanxcale.connector.kafka.sink.impl.LXWriterImpl:75)
[2020-03-31 09:33:45,604] INFO Writing 500 records (com.leanxcale.connector.kafka.sink.impl.LXWriterImpl:84)
[2020-03-31 09:33:45,910] INFO Commited session: 4 (com.leanxcale.connector.kafka.connection.impl.LXKiviConnectionProvider:78)
[2020-03-31 09:33:45,924] INFO Flushing records in writer for table com.leanxcale.connector.kafka.utils.metadata.TableId@a25226fa with name mytopic (com.leanxcale.connector.kafka.sink.impl.LXWriterImpl:54)
[2020-03-31 09:33:45,924] INFO Connecting to database... (com.leanxcale.connector.kafka.connection.impl.AbstractLXConnectionProvider:36)
[2020-03-31 09:33:45,924] INFO Attempting to open connection (com.leanxcale.connector.kafka.connection.impl.AbstractLXConnectionProvider:60)
[2020-03-31 09:33:45,924] INFO New session created: 5 (com.leanxcale.connector.kafka.connection.impl.LXKiviConnectionProvider:46)
[2020-03-31 09:33:45,924] INFO Table mytopic is already registered for the connector (com.leanxcale.connector.kafka.sink.impl.LXWriterImpl:75)
[2020-03-31 09:33:45,924] INFO Writing 500 records (com.leanxcale.connector.kafka.sink.impl.LXWriterImpl:84)
The lines represent:
-
The first connection to the DB.
-
How the connector does not find the table previously existing in the DB, so creates it based on the schema defined in the producer code.
-
How to insert the first record.
-
How, for the subsequent four records, the connector finds the table it previously created.
-
How to write these four records.
-
How it collects all 500 records, which is the default number of records to collect from the Kafka server during each poll call, and inserts these into LeanXcale.
1.3.5. Kafka Source Connector for LeanXcale
In order to run the Kafka Source connector for LeanXcale execute the following command:
nohup ./connect-standalone ../etc/kafka/connect-standalone.properties ../etc/kafka/connect-lx-sink.properties > nohup_connect_lx.out &
You can check in the log that the connector has started properly:
[2021-08-27 13:24:02,648] INFO Starting LX Source Task with config{connector.class=com.leanxcale.connector.kafka.LXSourceConnector, source.table=TABLE_TEST, tasks.max=1, topics=mytopic, source.from.mode=offset, source.poll.frequency=100, connection.properties=lx://localhost:9876/test@APP, value.converter.schema.registry.url=http://localhost:8081, fields.whitelist=trip_duration_secs,start_time,stop_time,start_station_id,start_station_name, start_station_latitude,start_station_longitude,end_station_id,end_station_name,end_station_latitude, end_station_longitude,bike_id,user_type,user_birth_year,user_gender, task.class=com.leanxcale.connector.kafka.source.LXSourceTask, name=local-lx-source, value.converter=io.confluent.connect.avro.AvroConverter, key.converter=io.confluent.connect.avro.AvroConverter, key.converter.schema.registry.url=http://localhost:8081, source.queue.size=11110, source.topic.name=${table}} (com.leanxcale.connector.kafka.source.LXSourceTask:47)
[2021-08-27 13:24:02,648] INFO Created connector local-lx-source (org.apache.kafka.connect.cli.ConnectStandalone:112)
[2021-08-27 13:24:02,649] INFO LXConnectorConfig values:
...
[2021-08-27 13:24:03,070] INFO Connecting to table:TABLE_TEST (com.leanxcale.connector.kafka.source.LXReader:85)
[2021-08-27 13:24:03,080] INFO LX Source Task properly started (com.leanxcale.connector.kafka.source.LXSourceTask:64)
[2021-08-27 13:24:03,081] INFO WorkerSourceTask{id=local-lx-source-0} Source task finished initialization and start (org.apache.kafka.connect.runtime.WorkerSourceTask:209)
[2021-08-27 13:24:03,186] INFO Built schema [Schema{STRUCT}] with fields [[Field{name=FIELD1, index=0, schema=Schema{INT32}}, Field{name=FIELD2, index=1, schema=Schema{STRING}}, Field{name=FIELD3, index=2, schema=Schema{FLOAT64}}]] for table:[TABLE_TEST] (com.leanxcale.connector.kafka.source.LXSourceTask:166)
The connector will automatically connect to the LX table and starting to streaming data to the kafka topic according to the given configuration.
If you set the log level to DEBUG you can check the details of the records sent to kafka in each poll request:
[2021-08-27 13:54:27,721] INFO Built schema [Schema{STRUCT}] with fields [[Field{name=FIELD1, index=0, schema=Schema{INT32}}, Field{name=FIELD2, index=1, schema=Schema{STRING}}, Field{name=FIELD3, index=2, schema=Schema{FLOAT64}}]] for table:[TABLE_TEST] (com.leanxcale.connector.kafka.source.LXSourceTask:167)
[2021-08-27 13:54:27,732] DEBUG Returning [660] records (com.leanxcale.connector.kafka.source.LXSourceTask:75)
[2021-08-27 13:54:28,863] DEBUG Returning [0] records (com.leanxcale.connector.kafka.source.LXSourceTask:75)
[2021-08-27 13:54:29,869] DEBUG Returning [0] records (com.leanxcale.connector.kafka.source.LXSourceTask:75)
...
[2021-08-27 13:54:43,935] DEBUG Returning [30] records (com.leanxcale.connector.kafka.source.LXSourceTask:75)
[2021-08-27 13:54:44,947] DEBUG Returning [50] records (com.leanxcale.connector.kafka.source.LXSourceTask:75)
[2021-08-27 13:54:45,959] DEBUG Returning [0] records (com.leanxcale.connector.kafka.source.LXSourceTask:75)
If you set the log level to TRACE you can also see the records details sent to kafka.
You can also start a kafka consumer to check the records received by the Kafka:
./kafka-avro-console-consumer --bootstrap-server localhost:9092 --topic TABLE_TEST --property schema.registry.url=http://localhost:8081
{"FIELD1":{"int":1000},"FIELD2":{"string":"field2 text:1000"},"FIELD3":{"double":1000.1}}
{"FIELD1":{"int":1001},"FIELD2":{"string":"field2 text:1001"},"FIELD3":{"double":1001.1}}
{"FIELD1":{"int":1002},"FIELD2":{"string":"field2 text:1002"},"FIELD3":{"double":1002.1}}
{"FIELD1":{"int":1003},"FIELD2":{"string":"field2 text:1003"},"FIELD3":{"double":1003.1}}
{"FIELD1":{"int":1004},"FIELD2":{"string":"field2 text:1004"},"FIELD3":{"double":1004.1}}
1.4. Kafka Examples
In this section you can find configuration examples for insert, update and delete. The section is based on the supplied configuration in the "Configuration" provision. Each of the following examples illustrate some configuration tips explained later.
1.4.1. Insert
To configure the connector to execute insert, just define insert as insert.mode.
name=local-lx-sink
connector.class=com.leanxcale.connector.kafka.LXSinkConnector
tasks.max=1
topics=mytopic
connection.url=lx://ip_bd_leanxcale:9876
connection.user=APP
connection.password=APP
connection.database=mytestdatabase
auto.create=false
insert.mode=insert
batch.size=1000
connection.check.timeout=20
key.converter=io.confluent.connect.avro.AvroConverter
key.converter.schema.registry.url=http://localhost:8081
value.converter=io.confluent.connect.avro.AvroConverter
value.converter.schema.registry.url=http://localhost:8081
sink.connection.mode=kivi
sink.transactional=true
sink.defer.timeout=10000
table.name.format=mytable
pk.mode=kafka
fields.whitelist=field1,field2,field3
This example configuration tips are the following:
-
auto.create: With a false value, the connector expects the target table to be already created. If not, it will raise an error.
-
insert.mode: If mode is insert, this is the operation used for insertion. It will fail if there is a duplicate PK.
-
batch.size: increased to 1000.
-
key.converter: Serializer of the registry key on the Kafka server, which is Avro for this example.
-
key.converter.schema.registry.url: URL where the schema registry is listening from and contains the configuration file.
-
value.converter: Serializer of the registry value in the Kafka server, which is also Avro, for this case.
-
value.converter.schema.registry.url: URL where you are listening to the schema registry. It is the one that contains your configuration file.
-
sink.connection.mode: LeanXcale connection mode value that defines the connection through the NoSQL interface. This is the only configuration available right now.
-
sink.transactional:
-
If false, means the ACID capabilities are not going to be complied. This scenario potentially increases the insertion performance, and is indicated in case of big initial loads without consistency requirements over operational environments.
-
If true, means the ACID capabilities are ensured. This is indicated in case of event driven architectures in which operational systems have to be aware immediately of changes to data and ACID must be complied. This decreases the performance of insertions but ensures coherency on transactions.
-
For more information about LeanXcale ACID transactions, please refer to Concepts / ACID transactions
-
-
table.name.format: Name of the target table. It can be defined with the same name as the topic or not. In this case, the target table name is "mytable".
-
pk.mode: Indicates the primary key mode of the target table. The kafka value means that it will use the kafka topic coordinates as pk in the target table. Please note it makes no sense to define pk.fields with this option. These coordinates are 3:
-
__connect_topic: name of the topic
-
__connect_partition: partition number on the topic
-
__connect_offset: offset number on the topic
-
The rest of the configurations can be checked in the default configuration described in the configuration section.
1.4.2. Upsert
To configure the connector to execute upsert, just define upsert as insert.mode.
name=local-lx-sink
connector.class=com.leanxcale.connector.kafka.LXSinkConnector
tasks.max=1
topics=mytopic
connection.url=lx://ip_bd_leanxcale:9876
connection.user=APP
connection.password=APP
connection.database=mytestdatabase
auto.create=true
insert.mode=upsert
batch.size=1000
connection.check.timeout=20
key.converter=io.confluent.connect.avro.AvroConverter
key.converter.schema.registry.url=http://localhost:8081
value.converter=io.confluent.connect.avro.AvroConverter
value.converter.schema.registry.url=http://localhost:8081
sink.connection.mode=kivi
sink.transactional=false
sink.defer.timeout=10000
table.name.format=${topic}
pk.mode=record_value
pk.fields=id
fields.whitelist=field1,field2,field3
This example configuration tips are the following:
-
insert.mode: If mode is upsert, this is the operation used for insertion. If there is a duplicate PK, it will overwrite it.
-
pk.mode: Indicates the primary key mode of the target table. The record_value value means that it will use the value field of the record stored in the topic.
-
pk.fields: If not defined, all the record value fields will be taken as pk. If defined, it will take the defined ones as pk. In this example, it will take the ID field.
1.4.3. Update
To configure the connector to execute upsert, just define update as insert.mode.
name=local-lx-sink
connector.class=com.leanxcale.connector.kafka.LXSinkConnector
tasks.max=1
topics=mytopic
connection.url=lx://ip_bd_leanxcale:9876
connection.user=APP
connection.password=APP
connection.database=mytestdatabase
auto.create=true
insert.mode=update
batch.size=1000
connection.check.timeout=20
key.converter=io.confluent.connect.avro.AvroConverter
key.converter.schema.registry.url=http://localhost:8081
value.converter=io.confluent.connect.avro.AvroConverter
value.converter.schema.registry.url=http://localhost:8081
sink.connection.mode=kivi
sink.transactional=false
sink.defer.timeout=10000
table.name.format=${topic}
pk.mode=record_key
fields.whitelist=field1,field2,field3
This example configuration tips are the following:
-
insert.mode: If mode is update, this is the operation used for insertion. Only works for duplicate PKs, it will overwrite it only if it exists. It will fail in other case.
-
pk.mode: If defined as record_key, it will use the key field value of the record stored in Kafka as the primary key in the target table. As there is no pk.fields defined in configuration, it will use all the fields contained in the record key as pk fields.
1.4.4. Delete
To configure the connector to delete rows based on the records receive, set delete.enabled as true.
name=local-lx-sink
connector.class=com.leanxcale.connector.kafka.LXSinkConnector
tasks.max=1
topics=mytopic
connection.url=lx://ip_bd_leanxcale:9876
connection.user=APP
connection.password=APP
connection.database=mytestdatabase
auto.create=true
insert.mode=insert
delete.enabled=true
batch.size=1000
connection.check.timeout=20
key.converter=io.confluent.connect.avro.AvroConverter
key.converter.schema.registry.url=http://localhost:8081
value.converter=io.confluent.connect.avro.AvroConverter
value.converter.schema.registry.url=http://localhost:8081
sink.connection.mode=kivi
sink.transactional=false
sink.defer.timeout=10000
table.name.format=${topic}
pk.mode=record_key
fields.whitelist=field1,field2,field3
This example configuration tips are the following:
-
delete.enabled: If deletion is or not enabled for this connector. If enabled, it is mandatory to set pk.mode as record_key. The connector will delete the record from the target table only if the record key contains a stored pk and the record value is null.
-
pk.mode: record_key mandatory if deletion is enabled.
1.5. Source Examples
1.5.1. Configuration example
Here you can find an example of source connector configuration (file connect-lx-source.properties)
name=local-lx-source
connector.class=com.leanxcale.connector.kafka.LXSourceConnector
tasks.max=1
key.converter=io.confluent.connect.avro.AvroConverter
key.converter.schema.registry.url=http://localhost:8081
value.converter=io.confluent.connect.avro.AvroConverter
value.converter.schema.registry.url=http://localhost:8081
connection.properties=lx://localhost:9876/test@APP
source.table=LX_SOURCE_TABLE
source.queue.size=10000
source.from.mode=offset
source.topic.name=${table}
source.fields.whitelist=field1,field2
In this example, the connector will connect to the table name LX_SOURCE_TABLE and will just copy the fields "field1" and "field2" of tuples inserted/modified after last run (offset stored in kafka) to a topic with the same name as the table
The possible values of the property source.from.mode are: * offset: tuples saved/updated after the offset returned by kafka. This is, the last tuple ts committed to kafka * now: only tuples inserted/updated from the starting ts will be returned * begin: all tuples, old and new ones, will be returned